Introduction
Swarm intelligence, a concept borrowed from nature, is revolutionizing the field of artificial intelligence (AI). The intricate patterns of coordination and collaboration seen in social insects like ants and bees have intrigued scientists for decades. Now, AI researchers are harnessing these natural phenomena to create smarter, more efficient algorithms and systems. In this article, we will delve into the fascinating world of swarm intelligence and explore how it is shaping the future of AI.12
The Essence of Swarm Intelligence
Swarm intelligence is a collective behavior exhibited by groups of simple entities, such as ants, bees, birds, or even fish, that interact with each other and their environment to achieve a common goal. Despite the individual entities’ simplicity, the group as a whole can display remarkably intelligent and adaptive behaviors. This concept has inspired AI researchers to develop algorithms and models that mimic the way these natural systems work.3
Key Characteristics of Swarm Intelligence
- Decentralization: In swarm intelligence, there is no central authority or decision-maker. Each entity operates independently based on local information and interactions with neighboring entities.
- Self-Organization: Swarm systems have a remarkable ability to self-organize. Through simple rules and local interactions, they can form complex patterns and solve intricate problems.
- Adaptability: Swarm systems can adapt to changing environments or circumstances. They exhibit robustness and resilience, ensuring the group’s functionality even in the face of individual entity failures.
Common Examples of Swarm Intelligence Algorithms
- Ant Colony Optimization (ACO): Inspired by the foraging behavior of ants, this algorithm is used for solving optimization problems like the traveling salesman problem. Ants deposit pheromones to communicate with other ants, reinforcing the paths that lead to better solutions.
- Particle Swarm Optimization (PSO): Modeled after the social behavior of birds or fish, PSO involves particles that move through a solution space, adjusting their positions based on their own best result and the best result achieved by their neighbors.
- Bacterial Foraging Optimization (BFO): This algorithm draws inspiration from the foraging behavior of bacteria. Bacteria move in a direction that optimizes their energy intake, and the algorithm simulates this behavior to find optimal solutions to complex problems.
- Firefly Algorithm: Based on the flashing patterns of fireflies, this algorithm models the attractiveness of solutions and the tendency of fireflies to move toward more attractive solutions.
- Swarm Robotics: Beyond optimization, swarm intelligence is also applied to robotics. In swarm robotics, a group of relatively simple robots collaboratively performs tasks that would be challenging for a single robot. Examples include environmental monitoring, search and rescue missions, and formation control.
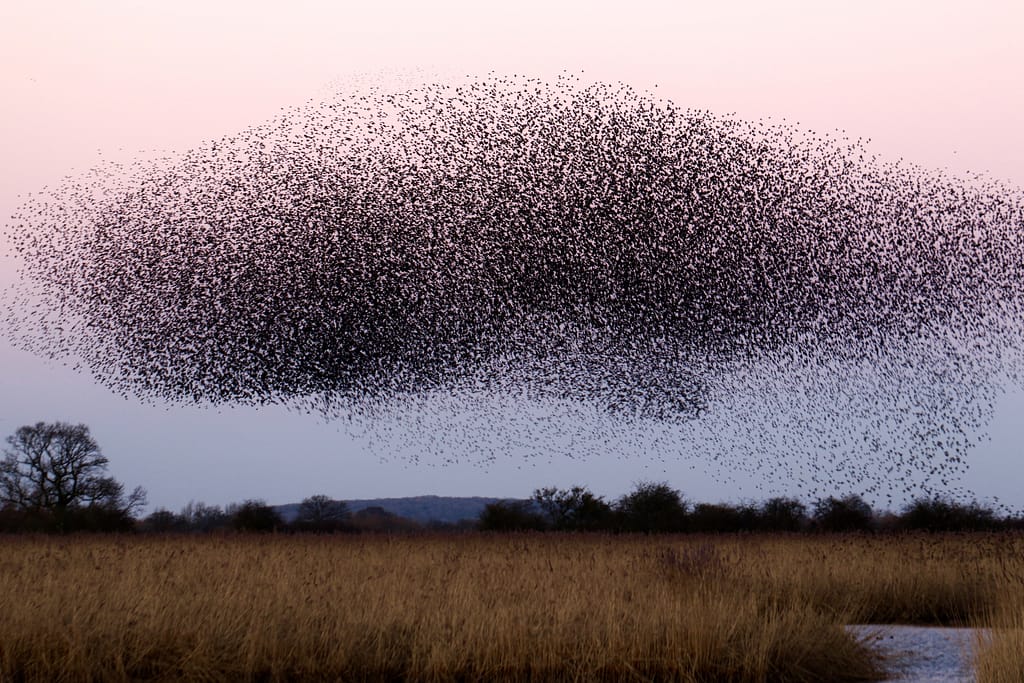
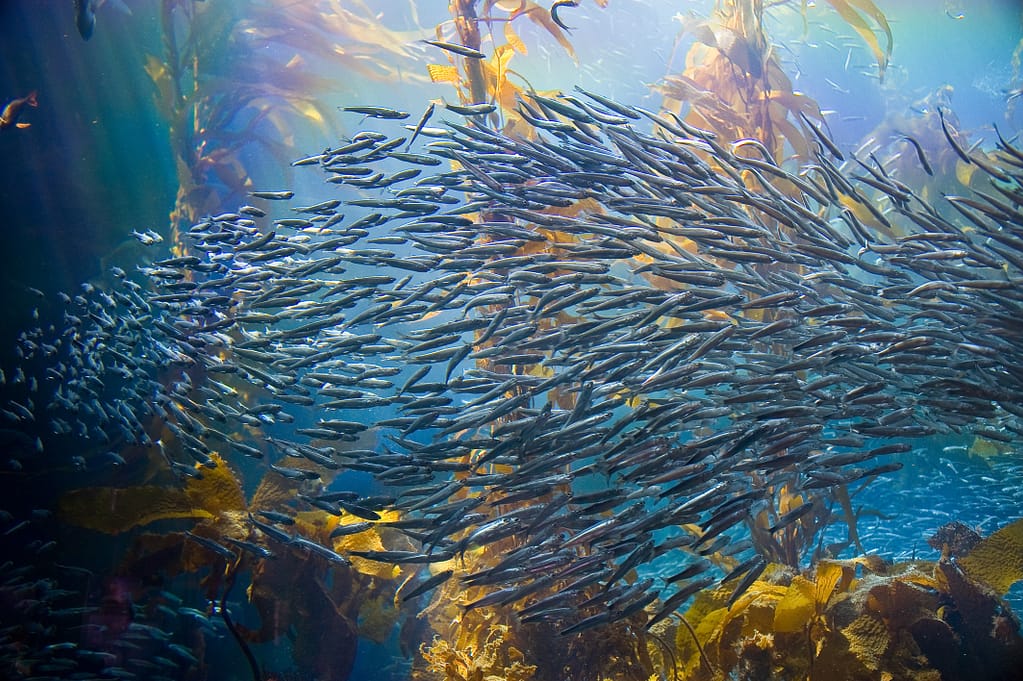
Swarm Intelligence in AI
AI researchers have recognized the potential of swarm intelligence to solve complex problems and optimize various tasks. Here are some areas where swarm intelligence is making a significant impact on AI:
- Optimization: Swarm intelligence algorithms, like particle swarm optimization (PSO) and ant colony optimization (ACO), are widely used for optimization problems. They can find optimal solutions to complex challenges, such as route planning, resource allocation, and parameter tuning.
- Robotics: Swarm robotics involves the coordination of multiple robots to accomplish tasks collectively. Inspired by social insects, these systems can be used in applications like environmental monitoring, search and rescue missions, and precision agriculture.
- Decision-Making: AI systems can employ swarm intelligence to make collective decisions based on the input from multiple sources. This can be applied in financial markets, traffic management, and disaster response.
- Data Clustering: Clustering algorithms based on swarm intelligence principles can efficiently group similar data points together. This is valuable in data analysis, pattern recognition, and recommendation systems.
- Network Routing: Inspired by ant foraging behavior, ACO algorithms4 are used in network routing to find the most efficient paths in communication networks.
The Future of Swarm Intelligence and AI
As AI continues to advance, the integration of swarm intelligence principles into machine learning models and algorithms is expected to grow. This combination holds the promise of creating AI systems that are not only intelligent but also adaptable, scalable, and robust.
Moreover, swarm-inspired AI has the potential to address some of the most pressing challenges in fields like healthcare, transportation, and environmental conservation. For instance, autonomous drones using swarm intelligence could be employed for disaster relief efforts, ensuring more effective and rapid response.
Conclusion
Swarm intelligence, drawn from the remarkable behaviors of social insects and other natural systems, is reshaping the landscape of artificial intelligence. Its decentralized, self-organizing, and adaptive nature makes it a valuable resource for solving complex problems and optimizing various tasks. As AI researchers continue to explore the possibilities of swarm intelligence, we can anticipate the development of AI systems that are more intelligent, efficient, and capable of tackling real-world challenges in innovative ways.